Asla Pitkanen
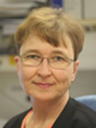
Special session on Big Data
Will talk about: Common Data Elements: A remedy for cure of underpowered preclinical studies
Due to many failures in translating the promising preclinical treatments into clinic, interest of industry in brain-related diseases is vanishing. This has raised a concern that there will be no novel, more efficient, and better tolerated treatments for many neurological and psychiatric diseases, including epilepsy. The problems in translation have been related to models used, differences in pathophysiology of the disease between species, and importantly, to lack of statistical power and reproducibility of pre-clinical studies. NIH has an initiative that has led to generation of common data elements (CDEs) for over 10 neurological diseases that can be used to harmonize clinical trials. Until recently, there have been very few attempts for harmonization of practices in pre-clinical studies. Epilepsy research community has initiated in 2013 an activity to generate CDEs for preclinical epilepsy research. In smaller scale, individual consortia like FP7 EPITARGET have established CDEs suitable for their experimental designs. Even though there is no experience yet, how the CDEs become applied in experimental laboratories, one can expect that their use will generate more accurate and reproducible large datasets, and reduce the concern related to underpowered pre-clinical studies.